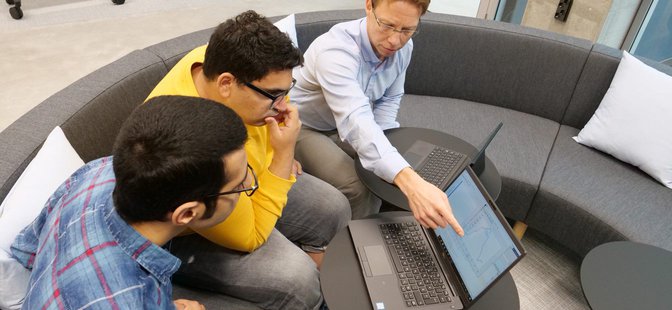
Computational Engineering and Analysis
The focus of the COMEA research group is in solution of interdisciplinary engineering problems by utilizing mathematical modeling and Computer Aided Engineering (CAE) methods.
The research work combines latest scientific advances, use of modern simulation software, and large-scale parallel computing for the solution of real-world problems. The group aims at bridging the gap between theory and practice such that it benefits both of the two worlds: Creation of new knowledge for engineering science and practical solutions that do work. High quality of the research output for this group is ensured by engaging in an active and scientifically rigorous publishing policy, and by a continuous collaboration with the industry. Within Turku University of Applied Sciences, the COMEA research group supports the other research groups by providing modeling and simulation expertise for their use.
Virtual prototypes and digital twins. Modern product design involves the use of CAE simulation, with realistic physics modeling, for ensuring product performance in the true operating environment. Carrying out product development using such virtual prototypes is significantly faster, cheaper and more flexible than using physical prototypes and experimental design alone. Moreover, the CAE simulation models from product development can later be extended for product operations. Together with online measurement data, this approach facilitates predictive maintenance, and aims at full system autonomy through digital twins.
Computational scenario analyses. There are many situations, in industry and beyond, in which carrying out measurements is prohibitively expensive, technically difficult or otherwise practically impossible – perhaps even hazardous. For such cases it is often possible to devise computational simulation models, which facilitate carrying out virtual experiments. Such mathematical models allow for investigation of different (even unforeseen or highly unlikely) scenarios, they help understand the underlying complex cause-effect relationships, and they facilitate informed and rigorous decision-making.
Modeling, simulation and optimization of complex systems. In practice, a device or a process (so-called system) is often composed of a large number of interconnected entities. Further, in practice, the behavior of such a system is seldom static. Instead, it is dynamically controlled and optimized depending the prevailing operating conditions. In the presence of myriads of different environments and use profiles, optimization of such complex systems (e.g. with respect to energy consumption) is only possible by using computational methods. But besides optimal solutions that can be transferred from the modeling software to physical control systems, this approach also yields a systemic understanding of the behavior of the complex system.
Frontier applications. Accurate modeling and simulation of new, complex or poorly understood phenomena is seldom directly possible with off-the-shelf software products. However, it is often possible to extend their modeling capacity to such frontier applications, either programmatically or by incorporating experimental measurement data in novel ways. Such tailored models, developed by using agile engineering and rapid proofs-of-concept, facilitate efficient description and analysis of the phenomenon in question.